|
|
Ivan Laptev
Visiting Professor at MBZUAI
on leave from INRIA Paris
Email: Ivan.Laptev -at- mbzuai.ac.ae
|
Short Bio:
Ivan Laptev is a visiting professor at MBZUAI on leave from INRIA Paris and a head of research at VisionLabs. He received a PhD degree in Computer Science from the Royal Institute of Technology in 2004 and a Habilitation degree from Ecole Normale Superieure in 2013.
Ivan's main research interests include visual recognition of human actions, objects and interactions, and more recently robotics. He has published over 150 technical papers most of which appeared in international journals and major peer-reviewed conferences of the field. He served as an associate editor of IJCV and TPAMI, he has served as a program chair for ICCV'23 and CVPR'18, he will serve as a program chair for ACCV'24 and is a regular area chair for CVPR, ICCV and ECCV. He has co-organized several tutorials, workshops and challenges at major computer vision conferences. He has also co-organized a series of INRIA summer schools on computer vision and machine learning (2010-2013) and Machines Can See summits (2017-2023). He received an ERC Starting Grant in 2012 and was awarded a Helmholtz prize in 2017.
Publications
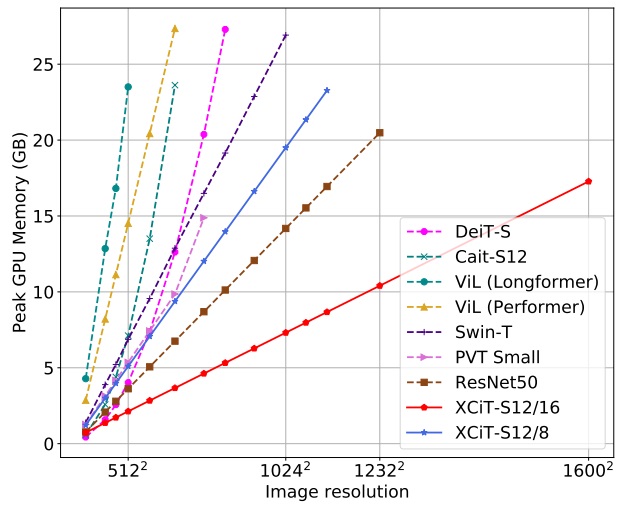 |
XCiT: Cross-Covariance Image Transformers (2021),
A. El-Nouby, H. Touvron, M. Caron, P. Bojanowski, M. Douze, A. Joulin, I. Laptev, N. Neverova, G. Synnaeve, J. Verbeek and H. Jégou;
in Proc. NeurIPS'21, virtual.
Project page
|
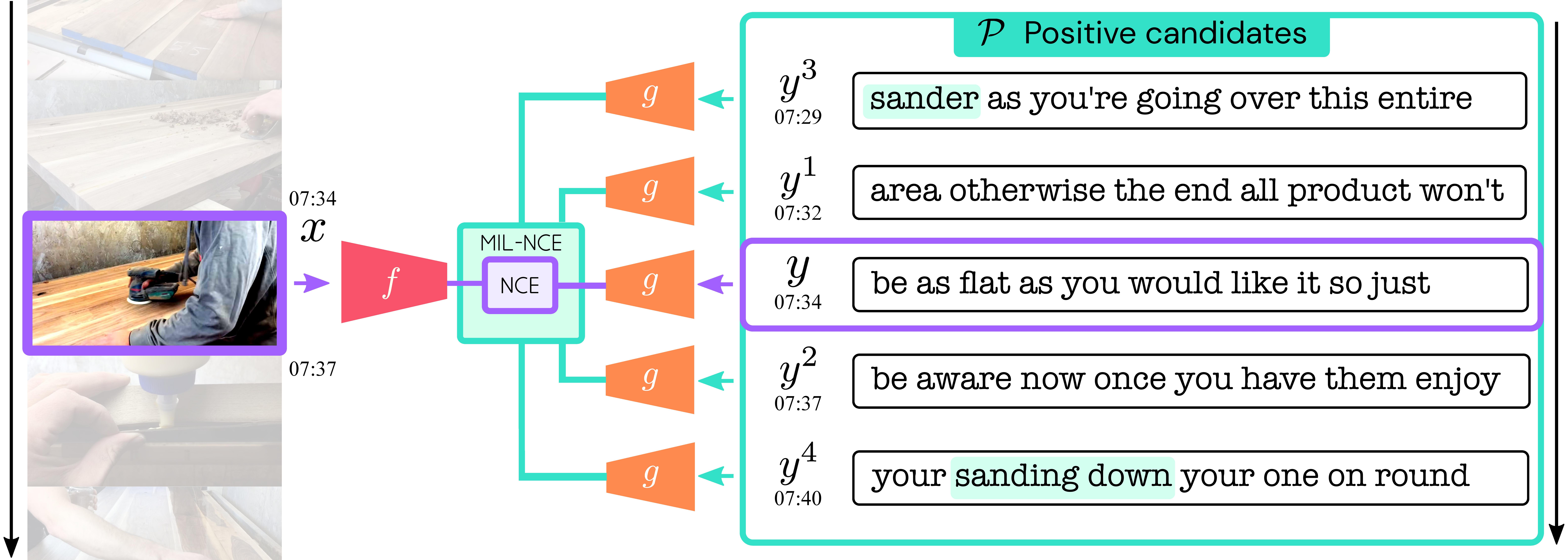 |
End-to-End Learning of Visual Representations from Uncurated Instructional Videos (2020),
A. Miech*, J.-B. Alayrac*, L. Smaira, I. Laptev, J. Sivic and A. Zisserman;
In Proc. CVPR'20, Seattle, WA, USA.
Project page,
YouCook2 zero-shot search demo,
I3D model,
S3D model.
|
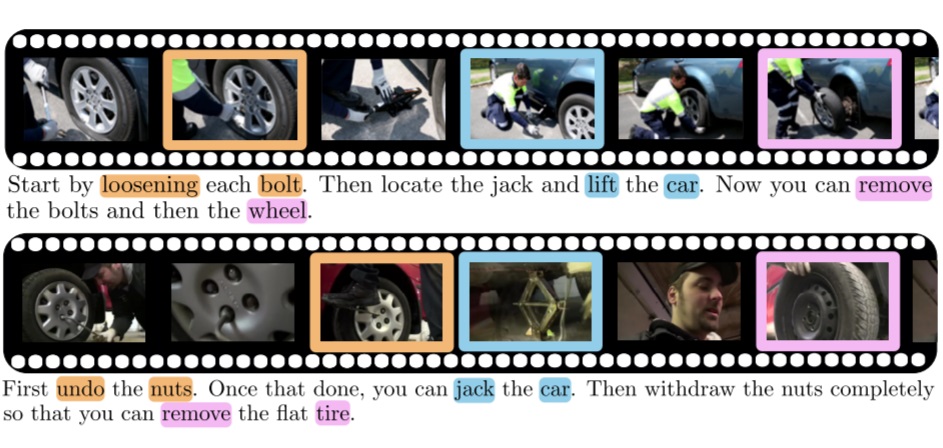 |
Unsupervised learning from narrated instruction videos (2016),
J.-B. Alayrac, P. Bojanowski, N. Agrawal, J. Sivic, I. Laptev and S. Lacoste-Julien;
in Proc. CVPR'16, Las Vegas, USA.
Project page
Extended version:
Learning from Narrated Instruction Videos (2017),
J.-B. Alayrac, P. Bojanowski, N. Agrawal, J. Sivic, I. Laptev and S. Lacoste-Julien;
in IEEE Trans. on Pattern Analysis and Machine Intelligence.
|
P-CNN: Pose-based CNN Features for Action Recognition (2015),
Project page
G. Chéron, I. Laptev and C. Schmid;
in Proc. ICCV'15, Santiago, Chile.
Context-aware CNNs for person head detection (2015),
Project page
T.-H. Vu, A. Osokin and I. Laptev;
in Proc. ICCV'15, Santiago, Chile.
Weakly-Supervised Alignment of Video With Text (2015),
P. Bojanowski, R. Lajugie, E. Grave, F. Bach, I. Laptev, J. Ponce and C. Schmid;
in Proc. ICCV'15, Santiago, Chile.
Unsupervised Object Discovery and Tracking in Video Collections (2015),
S. Kwak, M. Cho, I. Laptev, J. Ponce, and C. Schmid;
in Proc. ICCV'15, Santiago, Chile.
Is object localization for free? - Weakly-supervised learning with convolutional neural networks (2015),
Project page
M. Oquab, L. Bottou, I. Laptev and J. Sivic;
in Proc. CVPR'15, Boston, Massachusetts, USA.
On Pairwise Costs for Network Flow Multi-Object Tracking (2015),
Project page
V. Chari, S. Lacoste-Julien, I. Laptev and J. Sivic;
in Proc. CVPR'15, Boston, Massachusetts, USA.
Predicting Actions from Static Scenes (2014),
Project page
T.-H. Vu, C. Olsson, I. Laptev, A. Oliva and J. Sivic;
in Proc. ECCV'14, Zurich, Switzerland.
Weakly supervised action labeling in videos under ordering constraints (2014),
Project page
P. Bojanowski, R. Lajugie, F. Bach, I. Laptev, J. Ponce, C. Schmid and J. Sivic;
in Proc. ECCV'14, Zurich, Switzerland.
Learning and Transferring Mid-Level Image Representations using Convolutional Neural Networks (2014),
Project page
M. Oquab, L. Bottou, I. Laptev and J. Sivic;
in Proc. CVPR'14, Columbus, Ohio, USA.
Earlier version:
Technical Report HAL-00911179, Nov. 2013.
Efficient feature extraction, encoding and classification for action recognition (2014)
Project page
V. Kantorov, I. Laptev
in Proc. CVPR'14, Columbus, Ohio, USA.
Finding Actors and Actions in Movies (2013),
Project page
P. Bojanowski, F. Bach, I. Laptev, J. Ponce, C. Schmid and J. Sivic;
in Proc. ICCV'13, Sydney, Australia.
Pose Estimation and Segmentation of People in 3D Movies (2013),
Project page
G. Seguin K. Alahari, J. Sivic and I. Laptev;
in Proc. ICCV'13, Sydney, Australia.
Extended version:
Pose Estimation and Segmentation of Multiple People in Stereoscopic Movies (2015),
G. Seguin, K. Alahari, J. Sivic and I. Laptev;
in IEEE Trans. on Pattern Analysis and Machine Intelligence, 37(8):1643-1655.
Scene semantics from long-term observation of people (2012),
Project page
V. Delaitre, D.F. Fouhey, I. Laptev, J. Sivic, A. Gupta and A.A. Efros;
in Proc. ECCV'12, Florence, Italy.
People Watching: Human Actions as a Cue for Single-View Geometry (2012),
Project page
D.F. Fouhey, V. Delaitre, A. Gupta, A.A. Efros, I. Laptev and J. Sivic;
in Proc. ECCV'12, Florence, Italy.
Extended version:
People Watching: Human Actions as a Cue for Single View Geometry (2014),
D. Fouhey, V. Delaitre, A. Gupta, A. Efros, I. Laptev and J. Sivic;
in International Journal of Computer Vision, 110(3):259-274.
Object Detection Using Strongly-Supervised Deformable Part Models (2012),
Project page
H. Azizpour and I. Laptev;
in Proc. ECCV'12, Florence, Italy.
Actlets: A novel local representation for human action recognition in video (2012),
M.M. Ullah and I. Laptev;
in Proc. ICIP'12, Orlando, Florida, USA.
Learning person-object interactions for action recognition in still images (2011)
V. Delaitre, J. Sivic and I. Laptev;
in Proc. NIPS'11, Granada, Spain.
Density-aware person detection and tracking in crowds (2011),
Video,
Project page
M. Rodriguez, I. Laptev, J. Sivic and J.-Y. Audibert;
in Proc. ICCV'11, Barcelona, Spain.
Data-driven Crowd Analysis in Videos (2011),
Video,
Project page
M. Rodriguez, J. Sivic, I. Laptev and J.-Y. Audibert;
in Proc. ICCV'11, Barcelona, Spain.
Track to the Future: Spatio-temporal Video Segmentation with Long-range Motion Cues (2011),
Project page
J. Lezama, K. Alahari, J. Sivic and I. Laptev;
in Proc. CVPR'11, Colorado, US.
Semi-supervised learning of facial attributes in video (2010),
Project page
N. Cherniavsky, I. Laptev, J. Sivic, and A. Zisserman
in The First Int. Workshop on Parts and Attributes (in conjunction with ECCV 2010), Greece.
Recognizing human actions in still images: a study of bag-of-features and part-based representations (2010),
Project page
V. Delaitre, I. Laptev end J. Sivic
in Proc. BMVC'10, Aberystwyth, UK.
Improving Bag-of-Features Action Recognition with Non-local Cues (2010),
M.M. Ullah, S.N. Parizi and I. Laptev;
in Proc. BMVC'10, Aberystwyth, UK.
Automatic Annotation of Human Actions in Video (2009),
O. Duchenne, I. Laptev, J. Sivic, F. Bach and J. Ponce;
in Proc. ICCV'09, Kyoto, Japan.
Evaluation of local spatio-temporal features for action recognition (2009),
H. Wang, M. M. Ullah, A. Klaser, I. Laptev and C. Schmid;
in Proc. BMVC'09, London, UK.
Multi-View Synchronization of Human Actions and Dynamic Scenes (2009),
E. Dexter, P. Perez and I. Laptev;
in Proc. BMVC'09, London, UK.
Actions in Context (2009),
M. Marszałek, I. Laptev and C. Schmid;
in Proc. CVPR'09, Miami, US.
Modeling Image Context using Object Centered Grids (2009),
S.N. Parizi, I. Laptev and A.T. Targhi;
in Proc. DICTA'09, Melbourne, Australia.
Cross-View Action Recognition from Temporal Self-Similarities (2008),
I. Junejo, E. Dexter, I. Laptev and Patrick Perez;
in Proc. ECCV'08, Marseille, France.
Extended version:
View-Independent Action Recognition from Temporal Self-Similarities (2010),
I. Junejo, E. Dexter, I. Laptev and P. Perez;
in IEEE Trans. on Pattern Analysis and Machine Intelligence, 33(1):172-185.
Learning realistic human actions from movies (2008),
I. Laptev, M. Marszałek, C. Schmid and B. Rozenfeld;
in Proc. CVPR'08, Anchorage, US.
Retrieving actions in movies (2007),
I. Laptev and P. Perez;
in Proc. ICCV'07, Rio de Janeiro, Brazil.
Video Copy Detection: a Comparative Study (2007),
J. Law-To, L. Chen, A. Joly, I. Laptev, O. Buisson, V. Gouet-Brunet, N. Boujemaa and F.I. Stentiford;
in Proc. CIVR'07, Amsterdam, The Netherlands, pp. 371-378.
Improvements of Object Detection Using Boosted Histograms (2006),
I. Laptev;
in Proc. BMVC'06, Edinburgh, UK, pp. III:949-958.
Extended version:
Improving Object Detection with Boosted Histograms (2009),
I. Laptev; in Image and Vision Computing, vol. 27, issue 5, pp. 535-544.
Periodic Motion Detection and Segmentation via Approximate Sequence Alignment (2005),
I. Laptev, S.J. Belongie, P. Perez and J. Wills;
in Proc. ICCV'05, Bijing, China, pp. I:816-823.
Local Descriptors for Spatio-Temporal Recognition (2004),
I. Laptev and T. Lindeberg;
in ECCV Workshop "Spatial Coherence for Visual Motion Analysis",
Springer LNCS Vol.3667, pp. 91-103.
Extended version:
Local Velocity-Adapted Motion Events for Spatio-Temporal Recognition (2007),
I. Laptev, B. Caputo, C. Schuldt and T. Lindeberg;
in Computer Vision and Image Understanding, 108:207-229.
Velocity adaptation of space-time interest points (2004),
I. Laptev and T. Lindeberg;
in Proc. ICPR'04, Cambridge, UK, pp.I:52-56.
Galilean-diagonalized spatio-temporal interest operators (2004),
T. Lindeberg, A. Akbarzadeh and I. Laptev;
in Proc. ICPR'04, Cambridge, UK, pp.I:57-62.
Recognizing Human Actions: A Local SVM Approach (2004),
Christian Schuldt, Ivan Laptev and Barbara Caputo;
in Proc. ICPR'04, Cambridge, UK, pp.III:32--36.
Space-Time Interest Points (2003),
I. Laptev and T. Lindeberg;
in Proc. ICCV'03, Nice, France, pp.I:432-439.
Extended version:
On Space-Time Interest Points (2005),
I. Laptev;
in International Journal of Computer Vision, vol 64, number 2/3, pp.107-123.
Interest point detection and scale selection in space-time (2003),
I. Laptev and T. Lindeberg;
in Proc. Scale Space Methods in Computer Vision,
Isle of Skye, UK, Springer LNCS vol.2695, pp.372-387.
Velocity-adaptation of spatio-temporal receptive fields for direct
recognition of activities: An experimental study (2002),
I. Laptev and T. Lindeberg;
in Proc. ECCV'02 Workshop on Statistical Methods in
Video Processing, pp.61-66.
Extended version:
Velocity-adaptation of spatio-temporal receptive fields for direct
recognition of activities: An experimental study (2004),
I. Laptev and T. Lindeberg; in Image and Vision Computing 22:105-116.
Hand gesture recognition using multi-scale colour features, hierarchical models
and particle filtering (2002),
L. Bretzner, I. Laptev and T. Lindeberg;
in Proc. 5th IEEE International Conference on Automatic Face and Gesture Recognition,
Washington D.C., May, pp.423-428.
Extraction of linear objects from interferometric SAR data (2002),
O. Hellwich, I. Laptev and H. Mayer;
in Int. J. Remote Sensing 23(3):461-475, 2002.
A multi-scale feature likelihood map for direct evaluation of object hypotheses (2001),
I. Laptev and T. Lindeberg;
in Proc. IEEE Workshop on Scale-Space and Morphology, Vancouver, Canada,
Springer LNCS vol.2106, pp.98-110.
Extended version:
A distance measure and a feature likelihood map concept
for scale-invariant model matching (2003),
I. Laptev and T. Lindeberg; in International Journal of Computer Vision,
vol 52, number 2/3, pp 97-120.
Tracking of multi-state hand models using particle filtering
and a hierarchy of multi-scale image features (2001),
I. Laptev and T. Lindeberg;
in Proc. IEEE Workshop on Scale-Space and Morphology, Vancouver, Canada,
Springer LNCS vol.2106, pp.98-110.
Agilo robocuppers: Robocup team description (1999),
M. Klupsch, M. Luckenhaus, C. Zierl, I. Laptev, T. Bandlow, M. Grimme,
K. Kellerer, and F. Schwarzer;
in RoboCup-98: Robot Soccer World Cup II, Springer LNCS vol.1604, pp.446-451.
Multi-Scale and Snakes for Automatic Road Extraction (1998),
H. Mayer, I. Laptev, A. Baumgartner;
in Proc. ECCV'98, Freiburg, Germany,
Springer LNCS vol.1406, pp.720-733.
Extended version:
Automatic extraction of roads from aerial images based on scale-space and snakes (2000),
I. Laptev, H. Mayer, T. Lindeberg, W. Eckstein, C. Steger, and A. Baumgartner;
in Machine Vision and Applications 12(1):23-31.
Automatic Road Extraction Based on Multi-Scale Modelling, Context, and Snakes (1997),
H. Mayer, I. Laptev, A. Baumgartner, C. Steger;
in International Archives of Photogrammetry and Remote Sensing,
(32) 3-2W3 , pp.106-113
|
|