Paper

Data-driven Crowd Analysis in Videos
Proceedings of the IEEE International Conference on Computer Vision (2011), Oral.
PDF | Abstract | BibTeX Dataset
Abstract
In this work we present a new crowd analysis algorithm powered by behavior priors that are learned on a large database of crowd videos gathered from the Internet. The algorithm works by first learning a set of crowd behavior priors off-line. During testing, crowd patches are matched to the database and behavior priors are transferred. We adhere to the insight that despite the fact that the entire space of possible crowd behaviors is infinite, the space of distinguishable crowd motion patterns may not be all that large. For many individuals in a crowd, we are able to find analogous crowd patches in our database which contain similar patterns of behavior that can effectively act as priors to constrain the difficult task of tracking an individual in a crowd. Our algorithm is data-driven and, unlike some crowd characterization methods, does not require us to have seen the test video beforehand. It performs like state-of-the-art methods for tracking people having common crowd behaviors and outperforms the methods when the tracked individual behaves in an unusual way.BibTeX
@InProceedings{rodriguez11a,
author = "Rodriguez, M. and Sivic, J. and Laptev, I. and Audibert, J.-Y.",
title = "Data-driven Crowd Analysis in Videos",
booktitle = "Proceedings of the International Conference on
Computer Vision (ICCV)",
year = "2011",
}
Dataset
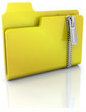
Data-driven Crowd Analysis Dataset
In order to perform data-driven analysis of crowd videos we aim to sample the set of crowd videos as broadly as possible. To this end, we have constructed our crowd video collection by crawling and downloading videos from search engines and stock footage websites. In addition to the large collection of crowd videos, the dataset contains ground-truth trajectories for 100 individuals, which were selected randomly from the set of all moving people. This dataset will be made publicly available soon.