Paper

Density-aware person detection and tracking in crowds
Proceedings of the IEEE International Conference on Computer Vision (2011), Poster.
PDF | Abstract | BibTeX
Abstract
We address the problem of person detection and tracking in crowded video scenes. While the detection of individual objects has been improved significantly over the recent years, crowd scenes remain particularly challenging for the detection and tracking tasks due to heavy occlusions, high person densities and significant variation in people's appearance. To address these challenges, we propose to leverage information on the global structure of the scene and to resolve all detections jointly. In particular, we explore constraints imposed by the crowd density and formulate person detection as the optimization of a joint energy function combining crowd density estimation and the localization of individual people. We demonstrate how the optimization of such an energy function significantly improves person detection and tracking in crowds. We validate our approach on a challenging video dataset of crowded scenes.BibTeX
@InProceedings{rodriguez11b,
author = "Rodriguez, M. and Sivic, J. and Laptev, I. and Audibert, J.-Y.",
title = "Density-aware person detection and tracking in crowds"
booktitle = "Proceedings of the International Conference on
Computer Vision (ICCV)",
year = "2011",
}
Dataset
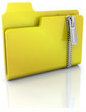
Crowd person detection dataset
This dataset consists of a diverse set of crowded videos. These videos contain considerable variation in terms of viewing angle, scale, crowd motion and crowd density. The dataset is divided into three annotated subsets. The first subset with 1200 annotated head bounding boxes was used to train a person detector. The second subset with all people annotated in 60 frames was used to train a density estimator. The last test subset with 1009 annotated head bounding boxes was deployed to evaluate the performance of the detection. This dataset will be made publicly available soon.